14:15 - 15:45
(When) Do Long Autoregressions Account for Neglected Changes in Parameters?
To construct forecasts for time series exhibiting breaks, the paper examines long autoregressions, where the number of lags is growing with T, and possible breaks are simply ignored.
Wer
Wo
To construct forecasts for time series exhibiting breaks, the paper examines long autoregressions, where the number of lags is growing with T, and possible breaks are simply ignored.
The paper shows that the OLS estimators are still elementwise consistent for the true autoregressive coefficients when neglecting a break in mean, but the sum of the estimators converges to unity. Thanks to this unit-root like-behavior of the fitted model, the resulting conditional forecasts are consistent for the true values.
As long as the dynamic structure is invariant, the robustness property of the forecasts holds a) under data-dependent lag length selection, b) for a piecewise smoothly varying mean function, and c) under general autoregressive dynamics of possibly infinite order including stationary long memory.
Under breaks in the dynamic structure, however, estimators are asymptotically biased, and the forecasts from long autoregressions are biased themselves even in the limit.
Ihr Kontakt
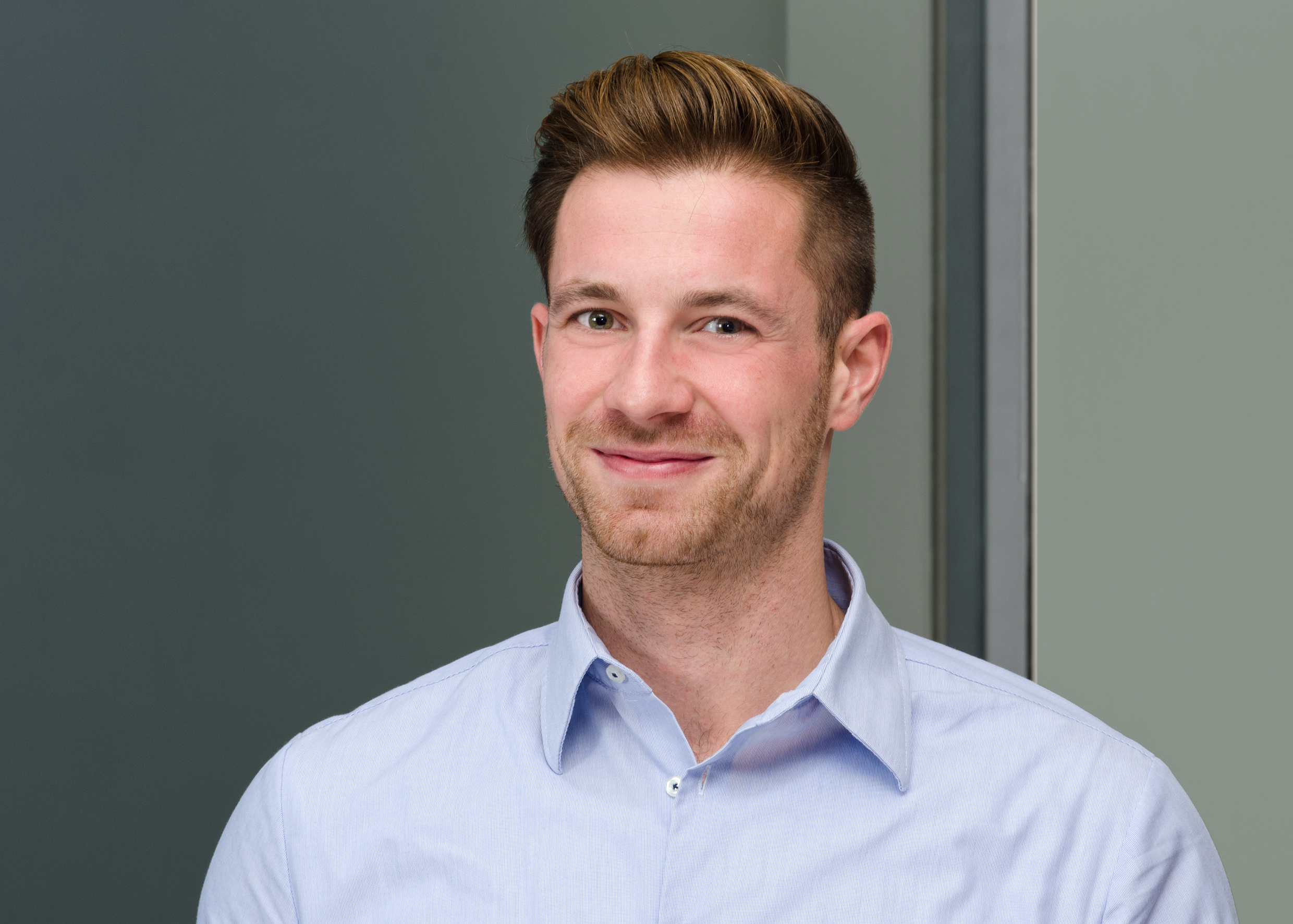
Wissenschaftlicher Mitarbeiter
Für Rückfragen stehe ich Ihnen gerne zur Verfügung.
+49 345 7753-865 Anfrage per E-Mail