Data Science in Financial Economics
The research group “Data Science in Financial Economics” focuses on developing and applying novel data science and machine learning methods in the field of financial economics. These methods are used to generate economic indicators from unstructured data, such as textual and imagery data, or web scraping. These indicators are then utilized in econometric analysis to address pertinent questions in financial economics.
Workpackage 1: Information frictions in financial markets
The asymmetric distribution of information is considered a key friction in economics. Different mechanisms aim to transfer information from the better informed to the less informed agent, where a question and answer (Q&A) setting is the most targeted form of information exchange. Employing a large language model approach, we seek to identify a lack of information content in answers to examine: How do markets react when they do not receive the expected quantity of information?
Newly developed methods are free of firm or financial specific context, and hence, applicable to Q&A outside the contextual domain of financial earnings conference calls. An example of another setting are projects in this work package in which we study the role of financial analysts as information intermediaries. Applying these generally methods reveals significant valuation effects, when obstructing the flow of information, either in calls or reports analysed by novel large language models.
Workpackage 2: Real estate market liquidity and macroprudential risk-shifting
Real estate markets are crucial as they are major repositories of household wealth and serve as collateral for mortgage loans, making their stability essential to financial systems. Our research leverages a novel data set - the European Real Estate Index (EREI) - to monitor liquidity and early indicators of price changes across European markets.
While Eurozone markets are influenced by a uniform monetary policy, they differ regionally in terms of their macroprudential policy regimes and economic factors. We explore how variations in national loan-to-value (LTV) policies affect bank lending, hypothesizing that simplistic LTV constraints may lead to suboptimal lending in less liquid markets.
Employing a difference-in-difference analysis, we assess the impacts of shifts in macroprudential policies on the quality of loan collateral, while also considering external factors such as geopolitical tensions and environmental challenges. Our aim is to enhance the understanding of the effects of macroprudential policies on lending practices and to integrate considerations of real estate market liquidity into broader financial stability considerations.
Workpackage 3: Adoption to climate change and limits thereof
We aim to understand how companies adapt to climate change and the inherent limitations of these adaptations. Utilizing Austrian ski regions as a laboratory, our study examines how ski areas implement climate change mitigation measures, such as artificial snow infrastructure. We identify these measures using satellite imagery and combine this with detailed firm level data.
Our research explores how affected regions, and the economic agents therein, collaborate to modify their local environments. We assess the impact of these modifications on employment and firm performance, and investigate how firm behaviour evolves when the limits of climate change adaptation are reached due to geographical constraints.
IWH Data Project: European Real Estate Index (EREI)
The IWH European Real Estate Database is a new dataset that illustrates the developments in the real estate market across Europe. The database includes monthly information, such as asking prices and listing details for sales and rentals, for 18 European countries between 2018 and 2024. A particular focus and novelty of the dataset is the possibility to assess real estate market liquidity, hence, the readiness with which assets can be sold within NUTS3 regions across major European economies.
Research Cluster
Financial Resilience and RegulationYour contact
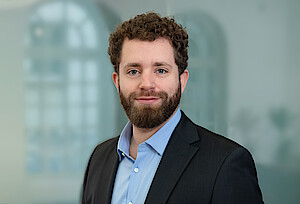
- Department Financial Markets
Refereed Publications
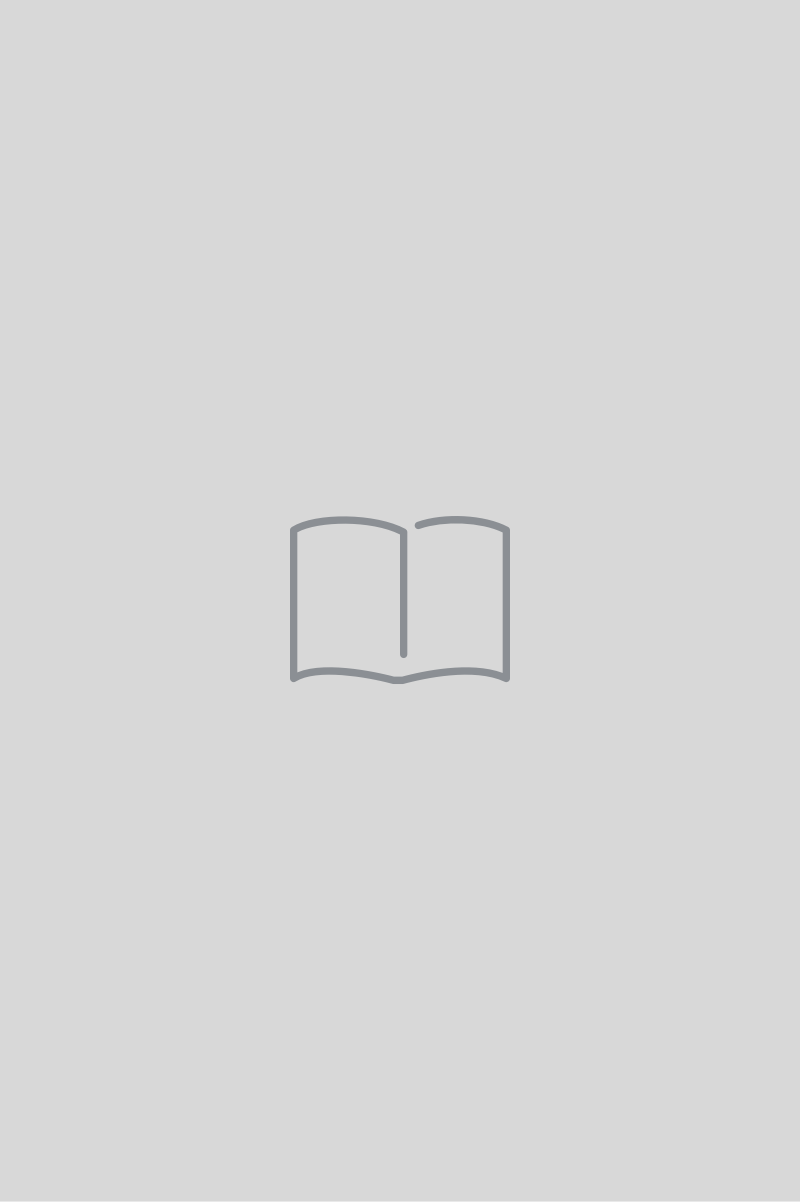
Understanding CSR Champions: A Machine Learning Approach
in: Annals of Operations Research, forthcoming
Abstract
In this paper, we study champions of corporate social responsibility (CSR) performance among the U.S. publicly traded firms and their common characteristics by utilizing machine learning algorithms to identify predictors of firms’ CSR activity. We contribute to the CSR and leadership determinants literature by introducing the first comprehensive framework for analyzing the factors associated with corporate engagement with socially responsible behaviors by grouping all relevant predictors into four broad categories: corporate governance, managerial incentives, leadership, and firm characteristics. We find that strong corporate governance characteristics, as manifested in board member heterogeneity and managerial incentives, are the top predictors of CSR performance. Our results suggest policy implications for providing incentives and fostering characteristics conducive to firms “doing good.”
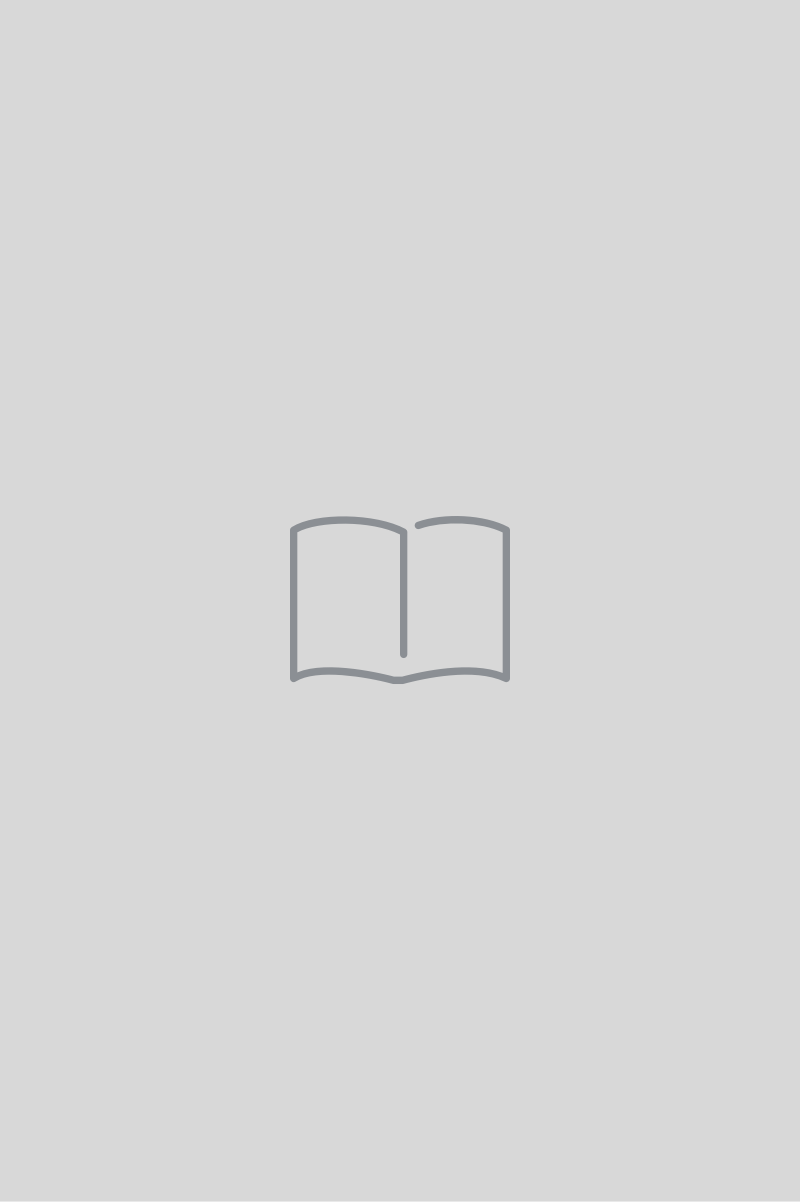
Asymmetric Reactions of Abnormal Audit Fees Jump to Credit Rating Changes
in: British Accounting Review, No. 2, 2024
Abstract
Considering the inherent stickiness of abnormal audit fees, our study contributes to the literature by decomposing abnormal audit fees into a jump component and long-run sticky component. We investigate whether and how changes in credit ratings asymmetrically affect the jump component of abnormal audit fees. We document a positive association between rating downgrades and the jump component. We find that heightened bankruptcy risk and misstatement risk are the mechanisms that drive this relationship. Further analysis shows that firms experiencing rating downgrades are more likely to receive a going concern opinion and experience longer audit report lags. Taken together, our findings provide direct evidence that credit ratings are significantly associated with abnormal audit fees, particularly with the jump component. Given the serial correlation of abnormal audit fees, our study sheds light on the importance of disaggregation of the abnormal audit fee residuals into the jump and long-run sticky components.
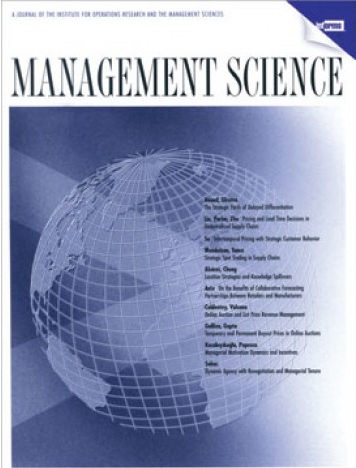
"Let Me Get Back to You" — A Machine Learning Approach to Measuring NonAnswers
in: Management Science, No. 10, 2023
Abstract
Using a supervised machine learning framework on a large training set of questions and answers, we identify 1,364 trigrams that signal nonanswers in earnings call questions and answers (Q&A). We show that this glossary has economic relevance by applying it to contemporaneous stock market reactions after earnings calls. Our findings suggest that obstructing the flow of information leads to significantly lower cumulative abnormal stock returns and higher implied volatility. As both our method and glossary are free of financial context, we believe that the measure is applicable to other fields with a Q&A setup outside the contextual domain of financial earnings conference calls.
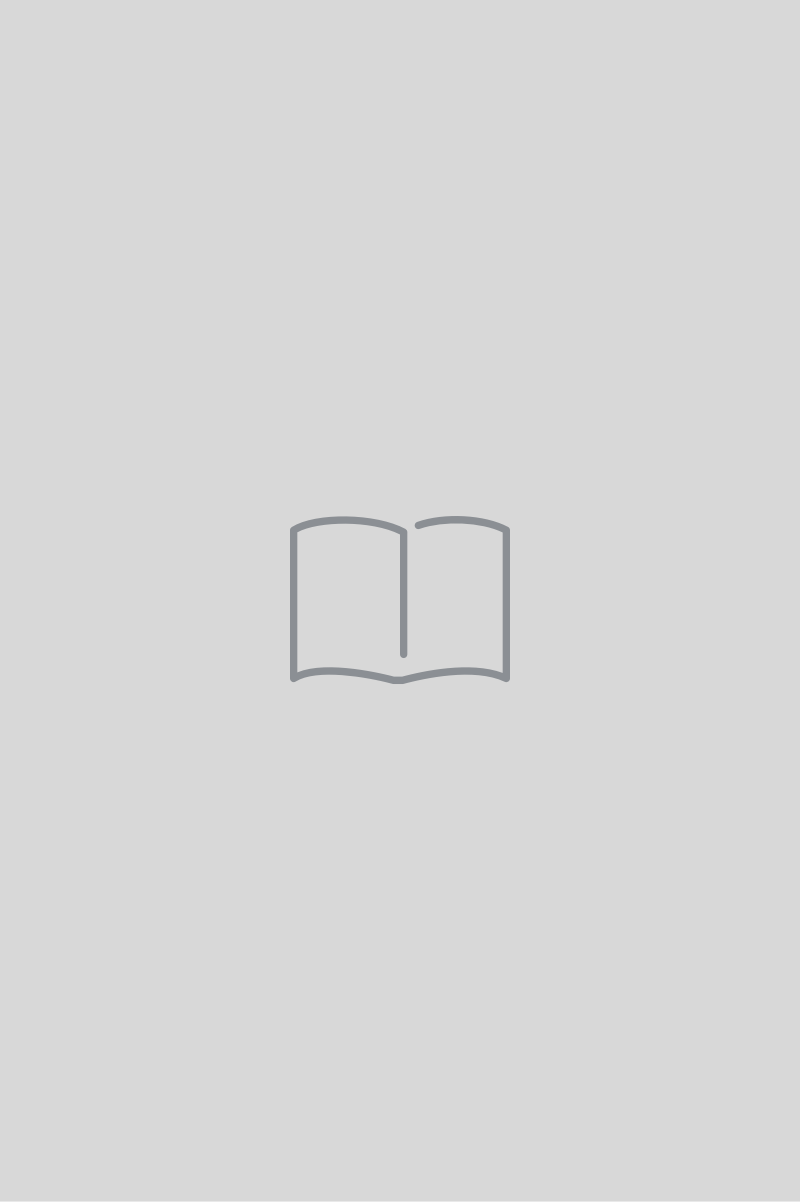
Exploring Accounting Research Topic Evolution: An Unsupervised Machine Learning Approach
in: Journal of International Accounting Research, No. 3, 2023
Abstract
This study explores the evolution of accounting research by utilizing an unsupervised machine learning approach. We aim to identify the latent topics of accounting from the 1980s up to 2018, the dynamics and emerging topics of accounting research, and the economic reasons behind those changes. First, based on 23,220 articles from 46 accounting journals, we identify 55 topics using the latent Dirichlet allocation model. To illustrate the connection between topics, we use HistCite to generate a citation map along a timeline. The citation clusters demonstrate the “tribalism” phenomenon in accounting research. We then implement the dynamic topic model to reveal the dynamics of topics to show changes in accounting research. The emerging research trends are identified from the topic analytics. We further explore the economic reasons and in-depth insights into the topic evolution, indicating the economic development embeddedness nature of accounting research.
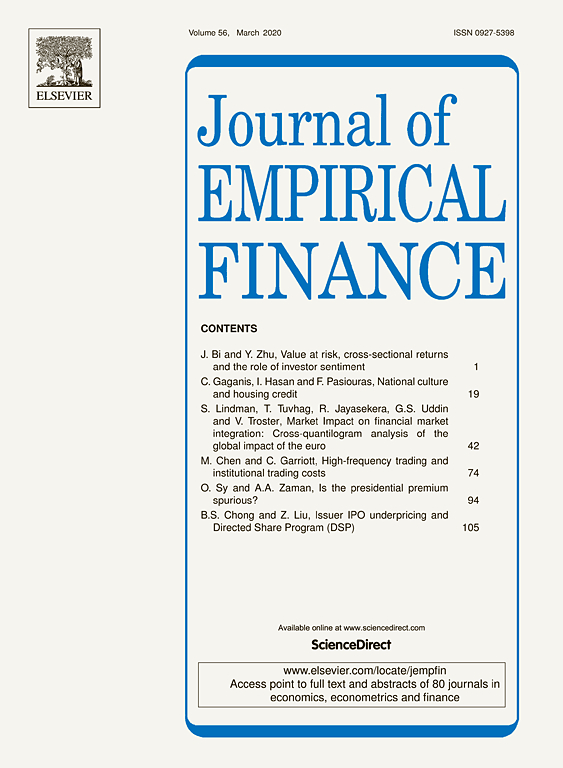
Herding Behavior and Systemic Risk in Global Stock Markets
in: Journal of Empirical Finance, September 2023
Abstract
This paper provides new evidence of herding due to non- and fundamental information in global equity markets. Using quantile regressions applied to daily data for 33 countries, we investigate herding during the Eurozone crisis, China’s market crash in 2015–2016, in the aftermath of the Brexit vote and during the Covid-19 Pandemic. We find significant evidence of herding driven by non-fundamental information in case of negative tail market conditions for most countries. This study also investigates the relationship between herding and systemic risk, suggesting that herding due to fundamentals increases when systemic risk increases more than when driven by non-fundamentals. Granger causality tests and Johansen’s vector error-correction model provide solid empirical evidence of a strong interrelationship between herding and systemic risk, entailing that herding behavior may be an ex-ante aspect of systemic risk, with a more relevant role played by herding based on fundamental information in increasing systemic risk.
Working Papers
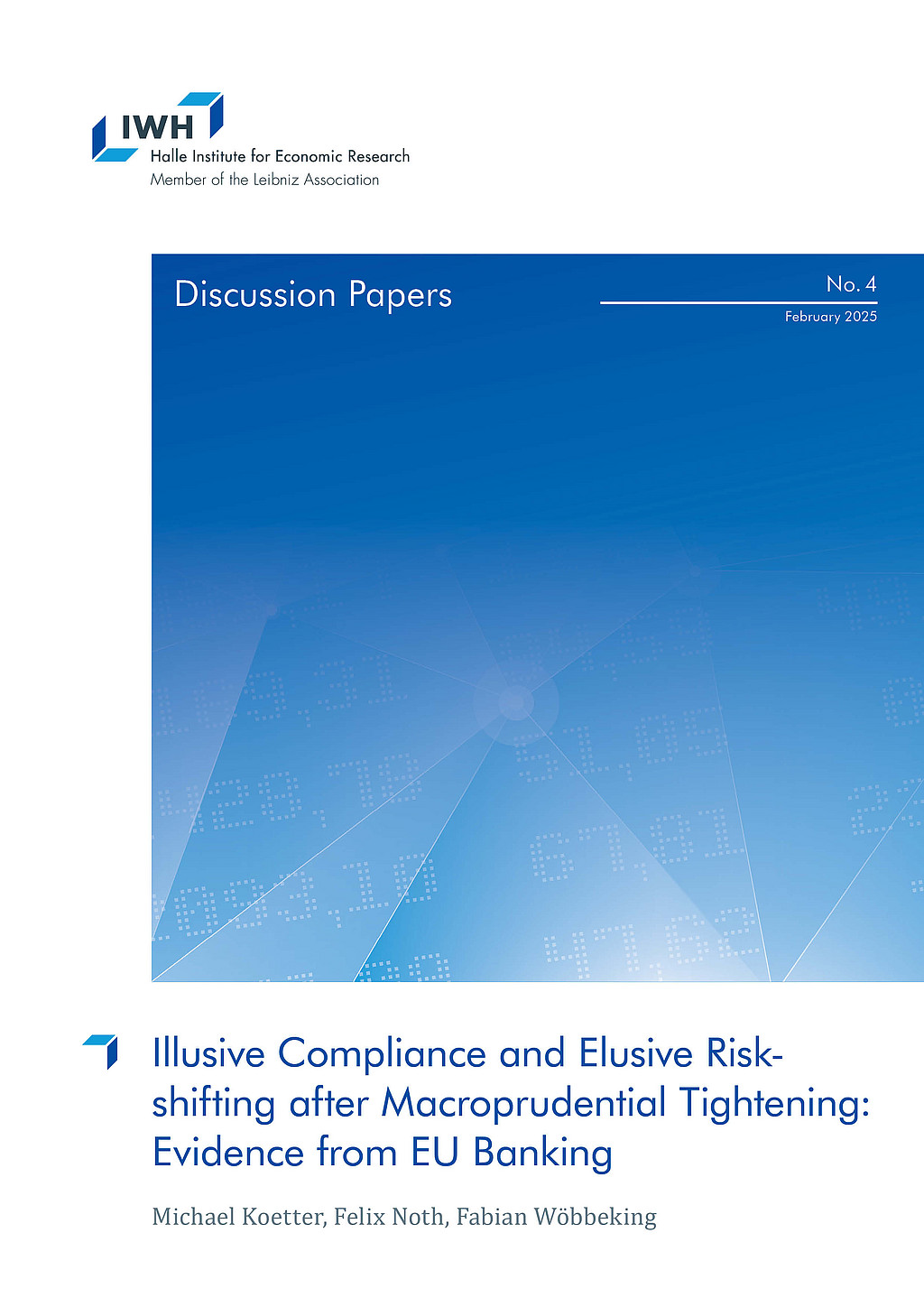
Illusive Compliance and Elusive Risk-shifting after Macroprudential Tightening: Evidence from EU Banking
in: IWH Discussion Papers, No. 4, 2025
Abstract
<p>We study whether and how EU banks comply with tighter macroprudential policy (MPP). Observing contractual details for more than one million securitized loans, we document an elusive risk-shifting response by EU banks in reaction to tighter loan-to-value (LTV) restrictions between 2009 and 2022. Our staggered difference-in-differences reveals that banks respond to these MPP measures at the portfolio level by issuing new loans after LTV shocks that are smaller, have shorter maturities, and show a higher collateral valuation while holding constant interest rates. Instead of contracting aggregate lending as intended by tighter MPP, banks increase the number and total volume of newly issued loans. Importantly, new loans finance especially properties in less liquid markets identified by a new European Real Estate Index (EREI), which we interpret as a novel, elusive form of risk-shifting.</p>
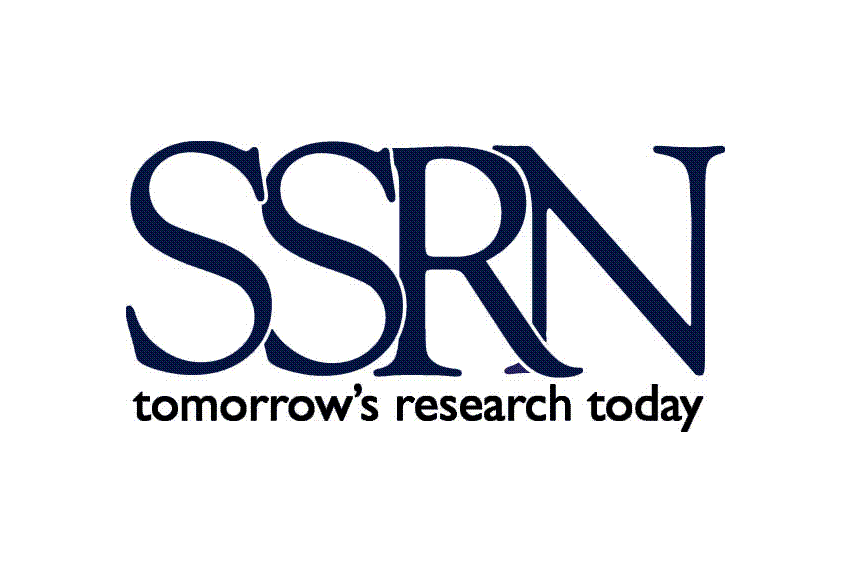
How to Talk Down Your Stock Performance
in: SSRN Discussion Papers, 2020
Abstract
We process the natural language of verbal firm disclosures in order to study the use of context specific language or jargon and its impact on financial performance. We observe that, within the Q&A of earnings conference calls, managers use less jargon in responses to tougher questions, and after a quarter of bad economic success. Moreover, markets interpret the lack of precise information as a bad signal: we find lower cumulative abnormal returns and a higher implied volatility following earnings calls where managers use less jargon. These results support the argument that context specific language or jargon helps to efficiently and precisely transfer information.